(Student Voices) The Topography of Data: Visualizing NEH Grants
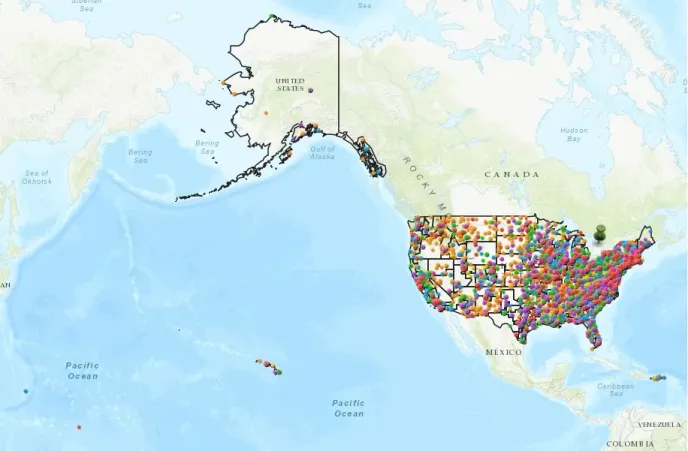
Screenshot of the interactive map the students created in ArcGIS.
This is the first of two blog posts written by graduate students at Carnegie Mellon University (CMU). In Fall 2020, the National Humanities Alliance partnered with a digital humanities course on three semester-long projects that the students worked on in groups. In this post, Ben Williams describes his experience working as part of the group tasked with creating a map of National Endowment for the Humanities (NEH) grants with a congressional district overlay for the NHA’s NEH for All initiative. This map combines several data sets into one visually accessible map and is an invaluable resource in highlighting the widespread impact of the NEH.
--
While enrolled in English 76-829, an introductory course to digital humanities at Carnegie Mellon University in the fall of 2020, our class collaborated with the National Humanities Alliance (NHA) on three digital humanities projects. My group’s task seemed simple: build a comprehensive map with a congressional district overlay that could communicate the impact of National Endowment for the Humanities (NEH) grant funding throughout the United States. In other words, we were asked to make the geographically widespread impact of the NEH more visually accessible for advocates. Our project was seemingly objective and its visual representation obvious, but over time we found ourselves having to continually reassess how we mapped data.
When we map, code, or quantify as humanists, questions arise about how power asymmetries might determine who or what counts. We thought deeply about not just the spatial spread of our data, but what I consider its topography. That is, the data’s layers built on humanistic contexts: robust stories, archived documents, and searchable details. Grants for exhibitions like “Picturing the Past” or “Adios Utopia: Dreams and Deceptions in Cuban Art Since 1950” were condensed to titles, geocodes, and program types. We knew little about their layered histories including their inception, implementation, and reception. Even within this relatively small data set, I was reminded of the need for what Tricia Wang calls “thick data,” 1 which, with gesture toward Clifford Geertz’s notion of “thick description,” 2 seeks to reveal “the social context of and connections between data points,” not just the “insights within a particular range of data points.” But to transform our data into thick data, we needed broad frames and minute details that might reveal the character of each of the data-funded grants, projects, and institutions captured in those Excel sheets and mitigate the issues of representation that come from staid data points.
We first had to sift through a surfeit of information about funding. And if there’s one thing I learned from looking at the collection of Excel sheets sent to us, it is that data overwhelms, and immense data overwhelms immensely. I found myself feeling adrift and incapable of contextualizing the information provided: unfamiliar names, unpredictably titled institutions, grants referred to by proxies or discrepant identifiers. Making sense of it required some investment in researching each of the programs in our data set. For example, we looked extensively at websites related to the “Muslim Journeys Bookshelf,” a collaboration between the American Library Association (ALA) and the NEH that provides public audiences in the U.S. with accessible and trustworthy materials related to Islam and Islamic civilizations. Rather than just seeing the geographic reach of the funding associated with this project, I started to understand the material and cultural impact of providing archival documents, books, films, and other programming resources to create space for audiences to become more familiar with people, places, history, faith, and cultures of Muslims around the world and in the US. Across testimonials, there was spirited reflection on the significance and positive reception of the content and materials from institutions and communities who now housed the bookshelf. Working through these details underscored the ways that feelings, thoughts, and reactions are often veiled by numeric data.
Through this additional research, the data started to become more humanistic and helped us see what and who we were trying to map. Eventually, with ArcGIS, a mapping software, the data’s topography was rendered over a spattering of colorful dots on the US with outlines of congressional districts. However, we found that as the data points were placed on the map, there were areas with dramatic absences, making the map appear partisan. While data points were sparse in red states and rural spaces, blue states and urban areas were filled with them. We determined that including data from Chronicling America, an NEH-sponsored repository of historic US newspaper information, would help visualize the reach of the NEH more evenly at different scales on the map. But the data was sent as a text file, which came with its own set of issues. Columns were indistinct and locations were hidden in parentheticals that made mapping difficult. Chris Warren, our digital humanities instructor, sifted through the data and used Pandas to compile it into a readable and, most importantly, usable form. Once put into ArcGIS, we saw the fruits of our labor scattered throughout the map, filling in the blank spots and underscoring the NEH-funded projects’ truer geographic distribution. We were given the code used to turn the text file of Chronicling America into something we could put on our map, and in this moment, I was reminded of how digital humanities works. It’s about templates of knowledge. Python, Regular Expressions, and Pandas are all useful tools and modes of knowledge that are easily transferable, and by availing ourselves to the code, we can put it to work when we encounter similar issues with messy data sets. So perhaps to revise an earlier comment, data does overwhelm, but less immensely when you have a template to help clean it.
Benjamin Williams is a Ph.D. student in Literary and Cultural Studies at Carnegie Mellon University. His research centers on how border rhetorics in literature, photography, and law construct conceptions of race, nation, and gender in the United States. He has presented his work examining migrant literature and visual culture at several conferences, including those hosted by Philosophy of the City, Comparative Drama, Northeast Modern Language Association (NeMLA), and Multi-Ethnic Literature of the United States (MELUS). He holds a B.A. in Philosophy and M.A. in English and American Literature from the University of Texas at El Paso.